Introduction
Biotech startups at the forefront of cutting-edge therapies often find their scientific innovation at odds with outdated operational tools. Consider a preclinical-stage company developing next-generation COVID treatments and T-cell therapies for solid tumors. While their R&D team breaks new ground scientifically, their day-to-day lab workflow relies on a patchwork of spreadsheets. Critical processes like drug batch traceability, mouse model tracking, sample lineage mapping, and quality control (QC) data entry are managed in Excel files shared via email. Collaboration among scientists is cumbersome, and preparing for clinical trials or audits means manually consolidating data across files.
This scenario isn't unique: even in 2025, many biotech labs still run on spreadsheets, creating hidden inefficiencies and risks. According to industry research, spreadsheet errors are alarmingly common, with one study finding that 94% of business spreadsheets contain critical errors that can affect decision-making processes. Additionally, around 20% of genetics papers have errors introduced by spreadsheet programs like Excel, particularly when handling gene names. These statistics highlight the cost of sticking with ad-hoc tools. As companies gear up for human trials and high-stakes partnerships, leadership faces a clear mandate: modernize the lab's operational stack or risk delays, errors, and compliance pitfalls.
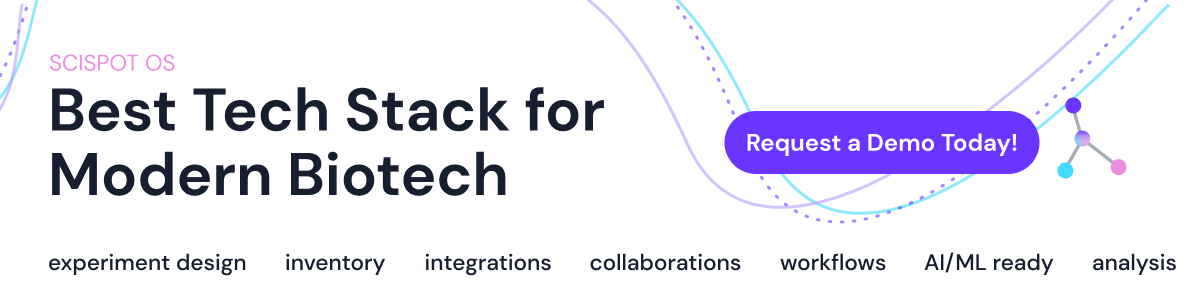
Operational Pain Points in a Preclinical Lab Environment
For biotech executives and lab managers, it's crucial to understand the specific workflow hurdles that a preclinical R&D lab experiences. The following operational pain points are common and costly when relying on spreadsheets and disconnected systems:
Drug Batch Traceability: Tracking experimental drug batches is difficult when data is scattered across files. There's no single view linking a batch to its formulation details, the experiments it was used in, and the outcomes. This makes it labor-intensive to answer questions like "Which batch was used in the mouse study showing an immune response anomaly?" Without a unified system, scientists must manually cross-reference lot numbers in multiple spreadsheets, increasing the risk of mix-ups or missing data.
Animal Model and Sample Tracking: Preclinical studies involve numerous mouse models and biological samples (tissues, cells, sera). Currently, each scientist maintains their own Excel sheet for tracking which mouse received which treatment, and how samples derived from these models are processed. This fragmented approach leads to version-control nightmares where two researchers might unknowingly use different spreadsheets with conflicting information. Mislabeling or lost lineage information can invalidate experiments. Manual record-keeping is error-prone, contributing to the high rate of lab errors from manual processes.
Sample Lineage Mapping: In advanced therapies, a single cell line or patient sample can spawn a chain of experiments and derivatives. Mapping this lineage via spreadsheets becomes nearly impossible as projects scale. Teams struggle to trace a data point (e.g., a flow cytometry result) back to the exact originating sample or protocol. The lack of an interconnected view of data slows down root-cause analysis and scientific decision-making.
Quality Control Data Silos: Quality control tests (for example, potency assays for T-cell products or sterility tests for batch releases) generate data that must be reviewed and signed off. Currently, QC results are stored in separate files apart from experimental data. This siloed storage means compiling a comprehensive QC report (say, for an IND regulatory filing or a partner audit) requires hunting through folders and emails. The process is not only inefficient but also risks overlooking a critical data point. Without real-time data tracking, issues in QC may be caught late, potentially derailing timelines.
Inefficient Multi-User Collaboration: Science is a team sport, but spreadsheets are single-player tools. Multiple researchers need to update project data simultaneously, something spreadsheets handle poorly. Shared online sheets offer limited relief; they often lack rigorous access controls, and there's no robust audit trail of who changed what and when. Collaboration via a modern lab platform, by contrast, would allow role-based permissions (e.g., a technician can input data but not alter protocols) and instant updates visible to all. The current setup forces scientists to spend extra hours coordinating changes, emailing updated files, or reconciling different versions. These are hours not spent on actual research.
Each of these pain points not only drains productivity but also poses strategic risks. If left unaddressed, they can result in significant delays (e.g., searching for data or redoing experiments), higher error rates, and compliance gaps that undermine progress toward clinical trials.

The High Cost of Inefficiency: Quantifying the Gap
How big is the efficiency gap when a lab relies on fragmented spreadsheets versus a modern integrated system? The difference is striking. Studies and case examples suggest that biotech labs can reclaim a great deal of lost time and quality by upgrading their digital infrastructure:
Time Spent vs Time Saved: Labs without streamlined data systems spend an inordinate amount of time on manual data handling. Data scientists and lab managers often report spending 50-80% of their time just collecting, cleaning, and organizing data rather than analyzing it. In preclinical workflows, this manifests as scientists spending evenings collating results from various files. By moving to a unified platform, labs have cut data processing time by approximately 70% through automation. For a startup, that could mean finishing in one day tasks that previously took a full workweek before a big meeting. Such acceleration isn't speculative; even large pharmaceutical R&D teams have achieved 40% faster progress to first-in-human trials by improving process and data flow, and greater gains are expected when AI and automation are fully integrated.
Manual Errors and Data Quality: Human error is a silent killer of R&D productivity. Mistyped entries, version confusion, or omitted data can force experiments to be repeated or, worse, lead teams down the wrong path. Traditional lab data practices (paper notebooks or Excel) are rife with these risks. Adopting an electronic, structured system can reduce error rates by 50-95% according to multiple industry sources. In one case, a preclinical CRO that replaced spreadsheets with a digital platform saw scientists achieve 3× better data quality (a dramatic drop in mistakes and inconsistencies). Fewer errors not only boost confidence in the science; they avoid costly rework and ensure that when data is presented to regulators or partners, it's trustworthy and reproducible.
Productivity and Operational Throughput: Consider the cumulative effect of daily inefficiencies. Researchers searching for the latest sample sheet or manually merging results for a report add up to substantial lost productivity. Automation and centralized data can cut routine labor hours by approximately 50%, effectively doubling the experimental output of the team without adding headcount. Tasks like generating a batch traceability report or an experiment summary, which might take a scientist half a day in Excel, can be completed in minutes with a query or automated dashboard. The reduction in repetitive tasks after implementing modern lab management systems can be as high as 85%. Freeing scientists from data wrangling yields a direct efficiency gain, potentially translating to completing 70% more studies per quarter than before, or moving a drug candidate into the next phase months sooner.
Hidden Costs of Non-Compliance: In highly regulated environments, operational inefficiency can incur regulatory costs. For example, if data isn't audit-ready, a biotech might face delays during an FDA inspection or due diligence by investors. Spreadsheets provide minimal audit trails, whereas a compliant lab system captures every action. In fact, companies that have transitioned to modern lab platforms report achieving significantly higher audit-ready compliance in their data management, meaning any data point can be traced with a full history, satisfying inspectors. This readiness avoids the scramble (and stress) of retrofitting compliance onto years of undocumented changes. It's hard to put a dollar value on peace of mind, but one can certainly quantify the risk reduction: avoiding a compliance failure or data-integrity issue that could cost the company its trial timeline or reputation.
In short, the efficiency gap between spreadsheet-driven labs and those with integrated digital platforms is on the order of tens of percent in lost time and quality. For a startup, closing this gap can mean reaching critical milestones (IND filings, partnership deals, clinical trial launches) significantly faster and with greater confidence. One rule of thumb: a 6-month delay in a drug program can equate to millions in additional burn rate and lost market opportunity. Thus, improving operational efficiency by even 50-70% is not just a process improvement; it's a strategic accelerant with real financial impact.

A Blueprint for a Next-Generation Lab Operating System
What would it take to solve these pain points and recapture the lost efficiency? The answer is a modern lab operating system, a unified platform purpose-built for life science R&D. Unlike a patchwork of LIMS (Laboratory Information Management System), ELN (Electronic Lab Notebook), and inventory trackers (or the manual equivalent thereof), a lab operating system is a single digital environment that connects every facet of lab work. Solutions like Scispot have emerged as category-defining options in this space, offering comprehensive platforms that address the needs of modern biotech companies. The blueprint for such a system includes several core components:
Graph-Based, Unified Data Model: At the heart of the platform is a graph database that interconnects projects, experiments, samples, animal models, drug batches, and results in a web of relationships. This is a game-changer for traceability. Instead of data living in isolated tables or files, everything is linked as a knowledge graph. One can traverse from a given mouse ID to the cell line it received, the batch of therapy administered, and the outcome measurements, all in a few clicks. This graphical ontology of lab data provides end-to-end context and enhances traceability by design. For research teams, that means instantly mapping the lineage of every sample or the usage history of every drug lot. Such a model not only prevents things from falling through the cracks, it also enables advanced queries (e.g., "find all experiments where Batch #202 had purity issues and link to the efficacy results"). In essence, the lab's data becomes a searchable, connected knowledge network rather than a filing cabinet of spreadsheets.
Real-Time Collaboration with Role-Based Access: A next-gen lab system is built for multi-user, real-time collaboration. Scientists can work concurrently on the platform with changes updating live, which is critical for fast-paced R&D. Just as importantly, the system provides role-based permissions and audit trails. Every user action (data entry, protocol edit, approval signature) is logged, supporting compliance requirements like FDA 21 CFR Part 11 for electronic records. Managers can assign roles (e.g., researcher, quality manager, admin) to ensure people see and do only what they're permitted. For example, an external collaborator might view results but not the full raw data, or a junior analyst might draft an experiment which a senior scientist then electronically signs. This controlled collaboration maintains data integrity and security while eliminating the version chaos of email attachments. The team would be able to collaborate on one source of truth, with confidence that every result is attributable and every change traceable. In effect, the platform becomes a living lab notebook that the entire team can securely share.
Embedded AI and Analytics (Lab "Digital Brain"): Modern lab operations platforms go beyond data storage. They come with embedded AI and analytics tools that act like a digital co-pilot for scientists. Current approaches integrate AI at multiple levels: an embedded assistant that researchers can query in natural language for insights, an in-platform environment for advanced analysis and automation, and capabilities that leverage the lab's knowledge graph. This means a scientist could literally "talk to their data" using intelligent chat interfaces, asking, for example, "Compare the T-cell response in all experiments using Batch #202 in mice vs. rats" and get an answer drawing from the underlying data relationships. AI can also auto-flag anomalies or suggest next experiments by finding patterns humans might miss. These capabilities turn the lab's data repository into an active intelligence system. Notably, AI-driven analysis and automation have been shown to further improve R&D efficiency, on top of basic digitization. Labs using such tools have seen processing speed improve significantly for certain discovery tasks and timelines shrink by additional months. The integrated data analysis tools mean data science and biology live in one place, with no need to export data to separate analysis software. Overall, this digital intelligence layer helps biotech teams make sense of complex datasets quickly and accelerates the cycle of hypothesis to insight.
Secure, Compliant Cloud Infrastructure (North America-Based): Given the sensitive nature of biotech data (proprietary science, patient-derived materials, potential national security projects), the platform must provide rock-solid security and compliance. Modern lab operating systems are deployed on cloud servers in North America, ensuring data residency for companies that require it (for example, a U.S. military collaboration would mandate data stays on domestic servers). The platforms are built with compliance in mind: they adhere to SOC2 standards for data security and FDA's CFR Part 11 for electronic records. Additionally, they support GxP (GLP for lab practices, GMP as they move to manufacturing) by maintaining complete audit trails and validation of data pipelines. In practice, this means companies can confidently use the system for regulated work. Every experiment record can be audited, electronic signatures capture approvals, and data integrity checks ensure nothing is tampered with. Encryption and access controls safeguard intellectual property and any patient data (with support for HIPAA, GDPR as needed). For executives, this compliance backbone reduces legal/regulatory risk dramatically. Regulatory readiness is "built-in." Importantly, a cloud solution also provides reliability and scalability (no downtime or data loss, robust backups). The North America hosting guarantees that if a company partners with U.S. government agencies or biotech firms, their data management meets jurisdictional requirements from day one.
Custom Onboarding and Rapid Implementation: One reason some labs hesitate to adopt new systems is the fear of long implementation times or rigid software that won't fit their unique processes. A modern lab operating system mitigates this with a highly configurable, no-code environment and "white-glove" onboarding support. Platforms like Scispot provide customizable templates for common workflows (e.g., cell culture logs, animal study protocols, assay result forms) but allow these to be tailored or new ones to be built without software coding. During onboarding, experts help migrate existing data (yes, even all those spreadsheets) into the platform's structured format, which can often be done in weeks, not months. The emphasis is on quick wins, getting teams up and running on the new system with minimal disruption. This is complemented by training and change management so that end-users are comfortable early. The result is rapid adoption; labs have reported that the system was fully in use and delivering value within a single quarter of kickoff. Furthermore, the platform is built to scale with the company: what works for a 10-person startup can easily handle a 100-person operation, with the addition of more projects, data volume, and integration of more lab instruments as needed. For biotech startups, this means they can invest in a solution now that will grow with them through clinical stages, avoiding the need to "rip and replace" down the line. In short, ease of implementation and flexibility ensure the technology adapts to the science, not vice versa.
Together, these elements form a holistic lab operations solution, effectively an operating system for biotech R&D. By adopting such a platform, a company transitions from a reactive, manual mode of operation to a proactive, automated one. It's the difference between driving with the headlights off and having a GPS with real-time traffic updates. Everything is connected, searchable, and analyzable, and compliance is assured in the background. Platforms like Scispot encapsulate this approach, which is why they're positioned as category-defining options for labs aiming to scale. However, the value of this blueprint isn't just in the technology itself, but in how it transforms the organization's capabilities moving forward.
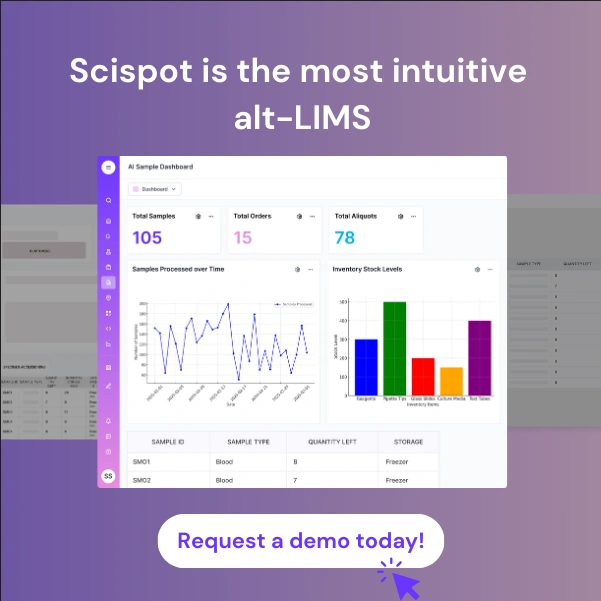
Strategic Implications: From Efficiency to Competitive Advantage
For biotech executives, implementing a next-generation lab platform is not merely an IT upgrade; it is a strategic move that can redefine the company's trajectory. Here's how an operational overhaul at a biotech startup translates into broader strategic benefits as it scales towards clinical trials:
1. Accelerated Path to Clinical Milestones: When R&D workflows are streamlined and data flows without friction, the entire research timeline compresses. Decisions can be made faster because data is readily available and reliable. In practical terms, a unified lab platform could shave months off the preclinical timeline by eliminating bottlenecks in data gathering and analysis. Hitting IND-enabling study goals sooner means earlier IND filing and potentially getting to Phase I trials ahead of schedule. In a competitive therapeutic area (like T-cell therapies for cancer), being first or faster can make a huge difference in securing partnerships and investor confidence. Moreover, faster cycle times allow the company to iterate more experiments in the same calendar time, increasing the chances of discovering a winning drug candidate. Industry analyses underscore this point; even large companies see a big financial upswing (hundreds of millions in NPV) by reducing time to clinic. For a startup, speed can mean survival and market leadership, and an efficient lab operation is a key lever to pull.
2. Enhanced Data Integrity and Regulatory Readiness: By instituting rigorous data practices early (GLP-grade data management, audit trails, version control), biotech startups build a foundation of trustworthiness. When the time comes to compile a regulatory submission or undergo an audit (whether by the FDA, a pharma partner, or a military research oversight), the company can confidently demonstrate that all results are backed by traceable records. This dramatically lowers the risk of compliance-related delays. For instance, if an FDA reviewer asks for the raw data behind an animal study, the team can produce an audit-logged record in minutes. By contrast, a spreadsheet-reliant lab might spend days scrambling to assemble and validate the same information. Proactively, having "audit-ready" systems 24/7 means the company can even invite external quality audits as a show of strength. This is particularly important for potential military collaborations or government grants where robust data security and compliance are often prerequisites for such partnerships. Strategically, a strong compliance posture enhances the company's reputation, turning operational excellence into a competitive advantage rather than a mere cost center.
3. Increased Team Productivity and Innovation Capacity: An often underestimated benefit of modernizing lab operations is its effect on people. Scientists who spend less time on drudgery (like copying data between sheets or troubleshooting inconsistencies) have more bandwidth for creative research and problem-solving. By eliminating up to 50% of manual labor through automation, the platform effectively gives the research team an "extra half" of their time back. Management can redirect this capacity towards more experiments, deeper data analysis, or exploring new therapeutic hypotheses. In other words, the company can do more science with the same headcount. This is crucial for a startup that must maximize output under tight budget constraints. Furthermore, an integrated platform breaks down silos between functions. Scientists, data analysts, QA personnel, and even external collaborators work off the same system, fostering a culture of transparency and collaboration. Over time, this can improve morale (no one enjoys wrestling with bad tools) and help attract talent. Top researchers are drawn to organizations where they can focus on science, not paperwork. The knowledge graph and AI tools embedded in the system also mean that new insights can emerge (via pattern recognition or simply easier data mining) that might otherwise be missed. In sum, a well-oiled digital lab frees the team to concentrate on innovation, which is the lifeblood of any biotech.
4. Better Decision Making and Project Management: With a consolidated view of operations, executives and project leaders gain real-time visibility into what's happening in the lab. Dashboards can show live status of all ongoing studies, inventory levels of critical reagents, or QC trends over time. This data-driven oversight enables proactive management: if a particular assay is consistently yielding variable results, it's flagged and addressed; if a project is behind schedule, resources can be reallocated early. Essentially, leadership moves from a reactive stance (learning about problems weeks later in a meeting) to a proactive one (getting alerts and insights from the system). This is about unlocking operational excellence using data to drive continuous improvement. The platform's intelligent assistant can even surface strategic recommendations, like suggesting which preclinical program has the best probability of success based on aggregated data, helping prioritize the portfolio. As biotech companies grow, this will be crucial for scaling. A single source of truth allows the company to manage complexity without losing agility. And when communicating with external stakeholders (Board members, investors, partners), the leadership can confidently provide up-to-date metrics and documentation, reinforcing credibility.
5. Readiness for Scale and Partnership: Lastly, adopting a robust lab operating system prepares the startup for its next chapters: clinical trials, partnerships, and even manufacturing. The data model can extend to capture clinical observations and patient sample data in early trials, maintaining continuity from lab to clinic. It also smooths tech transfer if the company partners with a larger pharma: having well-organized data and protocols makes it easier to hand off or integrate with a partner's systems. In the case of potential collaborations with the military or government, the fact that the platform is hosted on a secure North American cloud and meets high security standards will tick necessary boxes. As biodefense and biotech intersect, data security becomes part of national security, an arena where there is zero tolerance for breaches. By getting ahead on this front, the startup could become an attractive collaborator for sensitive projects because it can ensure data sovereignty and privacy. Moreover, scalability is about future-proofing: the chosen platform should be able to incorporate Good Manufacturing Practice (GMP) workflows when the therapy moves to production, and integrate with manufacturing execution systems (MES) or clinical data systems as needed. Essentially, the digital backbone laid in preclinical will carry the company through clinical development and even commercialization. Those who invest early in such infrastructure often find that their growth is less hindered by operational chaos. In contrast, competitors who delay may hit a wall; it's difficult to retrofit compliance and integration under the pressure of a Phase II trial or partnership due diligence. Thus, from an executive vantage point, implementing a system like Scispot's is a strategic insurance policy: it enables scale and makes the company more attractive for the partnerships and funding that fuel scale.
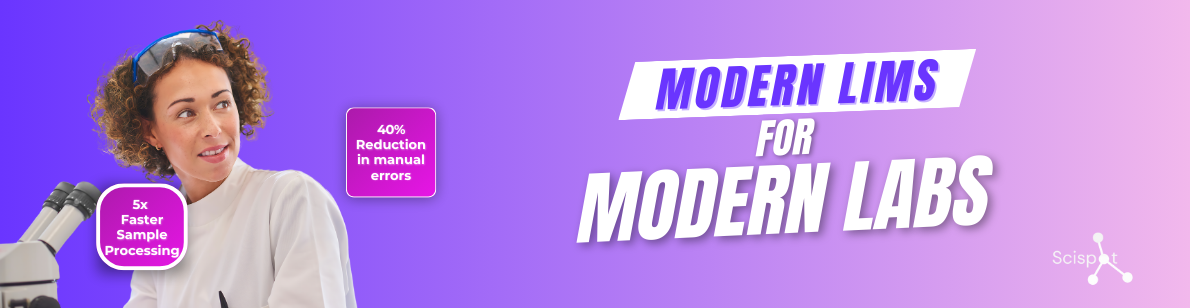
Conclusion: Key Takeaways for Biotech Lab Digital Transformation
For biotech executives and lab managers steering R&D organizations towards clinical success, the case is clear: operational excellence must accompany scientific excellence. Platforms like Scispot represent a new class of lab operating systems that can turn a hodgepodge of spreadsheets into a streamlined, intelligent engine for discovery. In summary, the key insights and takeaways from this analysis include:
Spreadsheets Exact a Steep Toll: Relying on manual, fragmented tools in a modern biotech lab leads to significant inefficiencies (lost time, duplication of work) and high error rates. Studies show spreadsheets used in business decision-making have a 94% chance of containing errors, and in the genetics field, approximately 20% of papers have errors introduced by spreadsheets. The operational drag can be quantified; companies can expect up to 70% faster project completion and significantly fewer errors by replacing spreadsheets with integrated solutions.
A Unified Lab Platform is a Force Multiplier: Implementing a graph-based, fully connected lab management system yields far more than incremental improvements. It provides end-to-end traceability (every sample, model, batch, and result linked) and real-time collaboration, which together eliminate data silos and communication gaps. The result is a lab that operates as a cohesive whole rather than isolated parts, boosting data quality and team productivity (e.g., one Scispot user saw a 50% reduction in labor hours immediately). This cohesion is crucial as R&D complexity grows.
Embedded AI = Accelerated Insights: The integration of AI and analytics (from automated data capture to intelligent assistants and integrated notebooks) turns the lab's data into actionable knowledge. Smart analytical tools can analyze data in seconds, surface trends, and even suggest next steps, amplifying the impact of human expertise. Early adopters of AI-driven lab systems position themselves to learn faster and make discoveries sooner, a decisive edge in fast-moving fields like immunotherapy.
Compliance and Security Are Non-Negotiable (and Achievable): Scaling a biotech without a strong data compliance framework is courting disaster. A modern lab platform enforces compliance (GLP, GMP, 21 CFR Part 11, etc.) by default through audit trails, permission controls, and validated workflows. It also provides enterprise-grade security (encryption, SOC2 compliance) and can ensure data residency (e.g., hosting on AWS in North America for sensitive projects). This means companies can be "inspection-ready" 24/7, dramatically reducing regulatory risks. In an era of increasing data regulations and cybersecurity concerns, having a secure, compliant infrastructure is as important as the science itself for long-term success.
Strategic Value: Faster, Smarter, More Competitive: Ultimately, investing in an operational platform like Scispot is a strategic decision that pays dividends in multiple ways. It accelerates the road to clinical trials, potentially reaching critical milestones 30-40% sooner. It improves decision-making through better data visibility and reliability. It enables the organization to scale seamlessly, whether that's doubling the R&D team or collaborating across company lines. And it converts operational excellence into a competitive advantage, attracting partners, investors, and talent by demonstrating that the company is not just scientifically savvy but also operationally world-class. In the high-stakes race of biotech R&D, those who build the right operational stack early will have a sustainable edge as they advance toward trials and, eventually, to patients.
In conclusion, the message to biotech labs is clear: the future belongs to those who marry great science with great systems. Adopting a lab operating platform is no longer a luxury or an afterthought; it's becoming the price of entry for serious contenders in next-generation therapeutics. For biotech startups, transitioning from spreadsheets to a scalable digital lab ecosystem will not only solve today's workflow headaches, it will set the foundation for tomorrow's breakthroughs, defining how biotech R&D is conducted as the industry marches toward an intelligent, data-centric future.
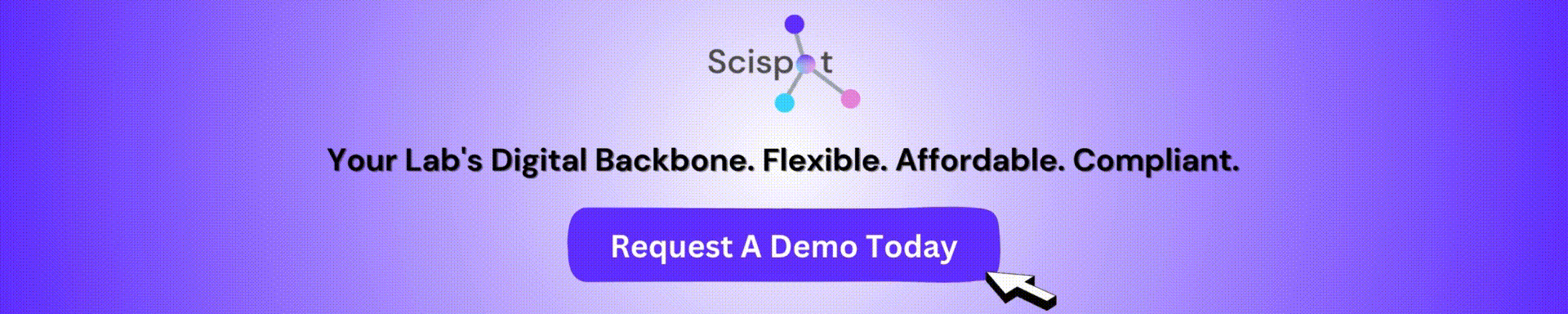