Life sciences laboratories are undergoing a transformation akin to the automotive industry's shift toward autonomous vehicles. Self-driving labs—highly automated research facilities leveraging artificial intelligence (AI) to design, execute, and analyze experiments autonomously—are poised to revolutionize research and development (R&D). Similar to how autonomous vehicles use sensors and AI to navigate roads, these labs utilize robotic instruments, precision sensors, and intelligent algorithms to manage complex experimental workflows independently.
Operating continuously, self-driving labs significantly reduce experiment turnaround times and operational costs compared to traditional laboratories. This shift allows scientists to focus more on strategic oversight, experimental design, and creative problem-solving. According to the U.S. Department of Energy (DOE), robotic self-driving labs can substantially lower costs while exponentially increasing throughput and data quality in scientific exploration, particularly in biotechnology, pharmaceuticals, and materials science.
This blog delves into the concept of self-driving labs, explores their unique business value for life science companies, highlights the AI advancements enabling their rise, presents real-world examples, discusses key challenges and mitigation strategies, and concludes with an outlook on how this transformative trend might shape biotech, pharmaceutical, and research institutions over the next decade.
What Are “Self-Driving” Labs?
Self-driving labs are highly autonomous research environments where AI drives experimental decision-making, and robotic instrumentation executes tasks. In these labs, AI software functions as the "brain," designing experimental plans, predicting outcomes, and adapting methodologies in real time, while robotics serve as the precise and tireless "hands" that carry out protocols.
This creates a closed-loop experimental cycle that iteratively refines experiments without manual intervention. For example, an AI algorithm might propose a drug candidate based on simulation data and then automatically direct robotic liquid handlers, incubators, and analytical devices to test the compound in cell assays. The AI immediately analyzes results, adjusts experimental parameters, and initiates subsequent tests, significantly shortening traditional research timelines. The DOE describes this autonomous workflow as enabling continuous validation, synthesis, and characterization of materials or biological entities, dramatically increasing research productivity.
Fundamentally, a self-driving lab operates similarly to a self-driving vehicle—utilizing real-time sensory data (instrument readings), advanced decision-making algorithms, and robotic actuators (pipettes, incubators, robotic arms) to achieve autonomous execution. Human scientists continue to set strategic goals and maintain oversight, but day-to-day experimentation runs independently, significantly accelerating discovery cycles.
Business Value Proposition for Life Science Labs
For biotech, pharmaceutical, and academic research organizations, adopting self-driving labs offers several compelling benefits:
1. Faster Experimentation Cycles
Self-driving labs drastically reduce research timelines by operating continuously without the constraints of human working hours or fatigue. This accelerates hypothesis testing and data turnaround from months to days or even hours. McKinsey reports that pharmaceutical companies could reduce their R&D cycle times by more than 500 days through comprehensive AI and automation implementation. For instance, Argonne National Laboratory's robotic system, Polybot, autonomously screened 90,000 material combinations in mere weeks, condensing what would typically require months of intensive human effort.
2. Greater Reproducibility and Reliability
Automated experimental execution minimizes human error, enhancing reproducibility and data reliability—critical in life sciences, which faces a well-documented reproducibility crisis. Robots provide consistent pipetting accuracy, precise reaction timings, and uniform protocol adherence. Detailed digital logging simplifies audits, quality control, and regulatory compliance, making self-driving labs particularly attractive to organizations pursuing rigorous regulatory approval pathways.
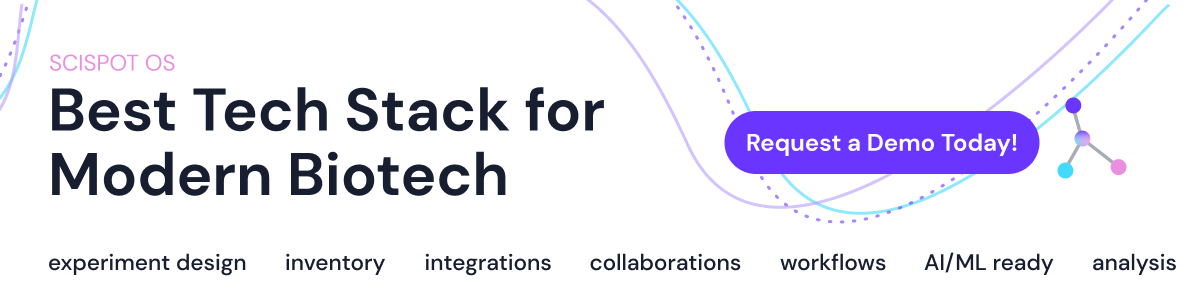
3. High-Throughput Screening at Scale
Self-driving labs enable unprecedented levels of experimental parallelization. Unlike traditional labs limited by manual handling and capacity constraints, automated facilities run multiple assays simultaneously, dramatically increasing throughput. This scalability allows for the screening of compounds in numbers far surpassing manual assays, moving from tens of thousands into millions in some cases. Such a scale fundamentally expands the breadth of experimentation, fueling innovation at an unprecedented pace.
4. Cost Reduction and Resource Optimization
While self-driving labs require upfront investment in automation and infrastructure, long-term cost savings are substantial. Automation reduces reliance on large, specialized human teams, allowing highly skilled scientists to concentrate on strategic tasks. Additionally, AI-driven optimal experimental designs minimize reagent use and efficiently utilize lab resources and equipment. McKinsey estimates that comprehensive automation and AI integration can cut overall R&D costs in pharma by approximately 25%. Merck KGaA's digital R&D initiatives exemplify this approach, utilizing an AI-driven Bayesian optimization platform ("BayBE") to streamline experiments, reduce costs, and accelerate innovation.
5. Democratization of Advanced R&D
AI-driven self-driving labs democratize sophisticated research capabilities by encapsulating expert knowledge, enabling smaller laboratories or organizations lacking specialist expertise to perform complex experiments. Open-source platforms further strengthen this democratization. For instance, Merck KGaA and the University of Toronto’s Acceleration Consortium have open-sourced their AI-based experiment planner BayBE to democratize access to cutting-edge AI tools, leveling the playing field and empowering broader scientific innovation.
As the adoption of AI-driven self-driving labs accelerates, life science organizations across biotech, pharma, and academia stand to benefit significantly—in speed, reliability, scalability, cost-efficiency, and accessibility—reshaping the very landscape of scientific discovery.
How AI Technologies Enable the Self-Driving Lab Vision
Recent advancements in artificial intelligence (AI) are pivotal in realizing the concept of self-driving laboratories. Several AI-driven capabilities have converged to facilitate the end-to-end automation of scientific experimentation:
End-to-End Workflow Automation
Modern AI systems can manage the entire research workflow—from hypothesis generation to experimental design, execution, and documentation. For instance, Sakana AI's "AI Scientist" project has demonstrated an AI agent autonomously generating novel research ideas, writing the necessary code, running experiments, and summarizing the results in a manuscript without human intervention. This showcases how AI can integrate previously separate steps (planning → execution → analysis → reporting) into a seamless pipeline. In practical lab terms, an AI might read scientific literature to propose an experiment, control lab robots to perform it, analyze the resulting data, and then suggest the next experiment in a continuous loop.
Closed-Loop Experimentation
At the heart of self-driving labs is a closed-loop cycle where the AI learns from each experiment and decides on the next one, continuously refining approaches. Cutting-edge frameworks like DOLPHIN, developed by Fudan University and the Shanghai Artificial Intelligence Laboratory, embody this concept. DOLPHIN generates research ideas, performs experiments (often via simulations or autonomous lab equipment), evaluates the results, and feeds those findings back to generate better ideas in the next iteration. Such closed-loop systems can run many cycles rapidly, optimizing parameters or exploring hypotheses much faster than a human-led iteration process.
Real-Time Data Integration & Analysis
AI in self-driving labs acts as a relentless data analyst, integrating data from multiple instruments and experiments instantly. This capability allows the system to detect patterns or issues on the fly. For example, AI algorithms, including computer vision and sensor analysis, can evaluate experimental outcomes faster and more consistently—immediately analyzing microscope images or genomic readouts—and decide whether the results meet the goals or if another iteration is needed. In a self-driving lab, data doesn't sit idle; it's continuously informing the AI's next actions.
Cross-Disciplinary Simulation and Modeling
Advanced AI models, especially large "foundation models" and physics-based simulations, are now being used alongside physical experiments. Before a robot ever mixes chemicals or assays a compound, an AI might simulate numerous possibilities in silico. Generative AI models can propose molecular structures or gene designs likely to succeed, which are then tested in the lab, creating a synergy between computation and experimentation. A federal science report noted that AI models can simulate candidates with unprecedented speed and low cost, complementing physical experiments. This integration enables self-driving labs to leverage AI-predicted outcomes to focus on the most promising experiments rather than brute-force testing every option.
Overcoming Human Limitations in Scale and Speed
AI and automation together far exceed human throughput capabilities. Self-driving labs can orchestrate dozens or hundreds of experiments in parallel, track innumerable variables, and operate continuously. Humans, by contrast, require rest and can manage only a limited number of simultaneous tasks. An AI agent doesn't get tired; it can plan experiments overnight and have results ready by morning. Researchers involved in AI lab projects often highlight this 24/7 capability, noting that while human scientists need rest, an AI-guided lab can "think" around the clock, methodically checking results for replicability.
These capabilities are no longer theoretical—they are being demonstrated in real projects and tools, paving the way for a new era in scientific discovery.
Real-World AI Systems Driving Self-Driving Labs
Several AI-driven systems and agents are actively bringing the concept of self-driving laboratories to fruition. Below are notable examples (excluding traditional lab informatics like ELNs/LIMS):
Scispot AI (Scibot) – AI Lab Operating System
Scispot offers a "Lab Operating System" integrated with an AI lab assistant named Scibot. Scibot serves as an AI agent that interfaces with lab data and instruments through a conversational chat interface. Scientists can use natural language commands to design experiments and execute protocols, with Scibot translating those into automated workflows. For instance, a user could instruct Scibot to "prepare a cell culture experiment with 96-well plates and send it to the liquid handler," and the system will carry out all those steps directly. Under the hood, Scispot connects to various lab instruments (e.g., Tecan liquid handlers, sequencers) and software via APIs, acting as a central brain that orchestrates multi-step experiments across equipment. This enables even small biotech labs to achieve high-throughput automation by simply communicating their needs to the AI. Scibot also assists with data analysis (e.g., generating dose-response curves) and data retrieval, reducing the effort required to gain insights from experiments. In effect, Scispot’s AI agent bridges human scientists with complex lab automation—the scientist specifies what to do, and the AI determines how to execute it within the lab systems. This commercially available platform accelerates AI adoption in real labs, providing a user-friendly entry point to self-driving lab capabilities.
Coscientist – AI Chemist in the Lab
Coscientist is an AI system developed by a team at Carnegie Mellon University, led by Assistant Professor Gabe Gomes. This system autonomously conducted real-world chemistry experiments. Coscientist utilizes large language models (LLMs), including OpenAI's GPT-4 and Anthropic's Claude, to plan and execute experiments. Notably, it successfully designed and performed Nobel Prize-winning chemical reactions, such as palladium-catalyzed cross-couplings, without human intervention. In demonstrations, Coscientist read literature and protocols for Suzuki and Sonogashira coupling reactions, then correctly designed a lab procedure in under four minutes and executed it with robotic tools—achieving the desired reaction on the first attempt. This marked the first instance of a non-human intelligence planning and performing such complex syntheses autonomously. The work was published in Nature, highlighting the scientific validation of the approach. Coscientist's design includes internet-enabled literature search, automated coding and debugging to control lab hardware, and analytical checks. Its success illustrates how an AI agent can function as a "digital chemist," navigating both information and physical experimentation. The team reported that such AI-driven experimentation can significantly increase the pace of discovery and improve reproducibility in chemistry.
The AI Scientist – Autonomous Research Agent
The AI Scientist is a project that debuted in 2024, aiming to automate the entire scientific discovery process. Developed by Sakana AI in collaboration with academic researchers, it showcased an AI agent that independently proposed new machine learning research ideas, wrote code to test them, ran the experiments, and even authored its findings. Notably, the AI Scientist produced multiple novel research papers in the machine learning domain with minimal human input. It even incorporated an automated peer-review mechanism to refine its results. While this was demonstrated in a computational setting (AI designing AI algorithms), the framework is general: such an agent could, in theory, be connected to wet-lab experiments as well. The significance of The AI Scientist lies in its proof that an AI can traverse an entire research loop—from idea to experiment to analysis to publication—on its own. It points toward a future where AIs become active researchers in labs, not just tools. For life science companies, this suggests that some aspects of R&D, like routine assay development or optimization problems, might be delegated to AI agents capable of researching solutions much faster than humans. The project's success in getting an AI-generated paper accepted also underscores the improving quality of AI-driven research outcomes.
DOLPHIN – Closed-Loop Auto-Research Framework
DOLPHIN, developed by Shanghai AI Lab and Fudan University, is an academic prototype of a closed-loop, open-ended research agent. While not tied to a specific commercial lab, it's designed to automate the scientific method itself further. DOLPHIN employs multiple AI techniques to generate new hypotheses by reading papers and using a knowledge-driven idea generation module. It then automatically writes experimental code, debugs it, runs experiments, and analyzes results to produce new hypotheses. The researchers tested DOLPHIN on various computational experiments, like image classification tasks, and found it could continuously generate novel ideas and even achieve results on par with state-of-the-art algorithms in some cases. Essentially, DOLPHIN is an AI that self-directs a research project toward open-ended goals, improving itself with each experiment. The name suggests agility and intelligence in navigating the "research ocean." For life sciences, one can envision a similar AI reading vast biomedical literature, formulating a new multi-step experiment (e.g., proposing a combination therapy to test on cell lines), executing it through a robotic lab, and iterating. Projects like DOLPHIN are important because they hone the algorithms that will power more autonomous labs—including how to make AI's decision-making interpretable and how to determine when to stop or change course in an experiment.
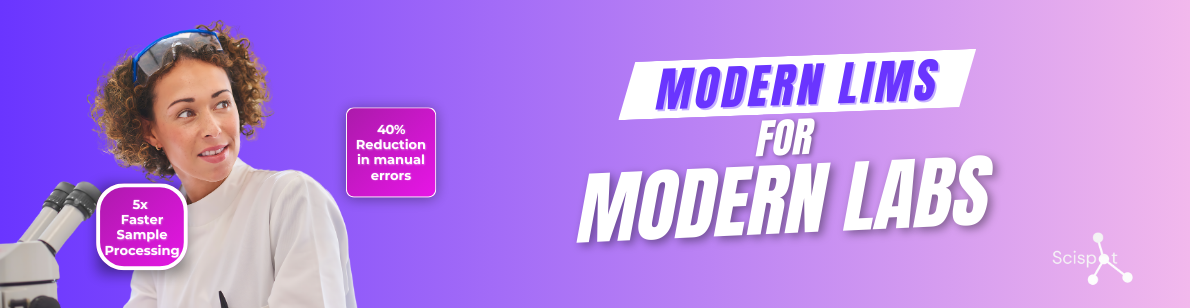
Other Notable AI Enablers
In addition to the previously discussed systems, a growing ecosystem of AI tools is contributing to the advancement of self-driving laboratories:
Bayesian Optimization Engines: Merck’s BayBE
Bayesian optimization engines assist in identifying the most promising experiments to conduct next. Merck KGaA, in collaboration with the Acceleration Consortium at the University of Toronto, developed the Bayesian Back End (BayBE), an AI-driven experimentation planner. Released as open-source software in December 2023 under the Apache 2.0 license, BayBE serves as a general-purpose toolbox for smart iterative experimentation, with a focus on chemistry and materials science. It provides recommendations for optimized experiments and can act as the "brain" for automated equipment, enabling entirely closed-loop self-driving laboratories. BayBE's capabilities include custom parameter encodings, multi-target support via Pareto optimization, active learning, and transfer learning, making it a versatile tool for various experimental campaigns.
Autonomous Discovery Platforms: LabGenius’s EVA™
LabGenius, a London-based biotechnology company, utilizes its proprietary platform, EVA™, to accelerate the discovery of novel therapeutic antibodies. EVA™ combines machine learning models with robotic automation to autonomously design, conduct, and learn from experiments. This closed-loop system enables the rapid identification of high-performing antibodies, including complex multispecific formats, by exploring large areas of antibody design space with minimal human bias. LabGenius has demonstrated the ability to design, produce, and characterize panels of up to 2,300 multispecific antibodies in just six weeks. The platform employs active learning methods, such as Multi-Objective Bayesian Optimization (MOBO), to efficiently co-optimize antibodies across multiple properties, including potency, efficacy, and developability. In 2024, LabGenius secured £35 million in Series B financing to enhance its platform further and advance its pipeline of multispecific antibodies toward clinical development.
Cloud Labs with AI Integration
Robotic cloud laboratory services, such as Strateos and Emerald Cloud Lab, provide remote-controlled automated lab facilities. When integrated with AI-driven design of experiments, these cloud labs enable a form of self-driving experimentation accessible on demand. Scientists can deploy AI algorithms to optimize reactions or cell assays remotely—the AI determines the experimental parameters, and the cloud-based robots execute the experiments. This emerging combination hints at "as-a-service" self-driving labs, allowing organizations without their own automation infrastructure to leverage AI for conducting autonomous experiments over the internet.
Each of these systems addresses different components of the self-driving lab paradigm, from intelligent experiment planning to physical execution. Collectively, they demonstrate that the technology is rapidly maturing: real laboratories are already employing AI agents to plan workflows, robotics to perform experiments, and closed-loop algorithms to refine results. However, alongside these promising developments, challenges remain to be addressed for widespread adoption.
Challenges and Risks
While the potential of AI-driven autonomous labs is significant, life science organizations must carefully navigate several critical challenges to ensure successful adoption:
1. Instruction Fidelity and Safety
Precision and reliability in experimental execution are essential. AI agents must precisely interpret complex experimental protocols and control laboratory equipment without error. Even minor mistakes—such as adding incorrect reagent volumes, incorrect incubation temperatures, or mis-timed reactions—can invalidate results or pose safety risks. For example, the Carnegie Mellon-developed AI system, Coscientist, encountered a coding error controlling a heating device during an automated chemical synthesis. Remarkably, the AI autonomously detected and corrected this mistake by referencing the equipment’s manual in real time, highlighting both the risks and the potential of automated error detection systems1.
To mitigate such risks, rigorous validation of AI-generated commands and comprehensive fallback safety mechanisms are crucial. Labs adopting these technologies must implement strict constraints—such as upper and lower bounds for reagent quantities or temperatures—that the AI cannot override. Moreover, protocols often contain nuanced, tacit knowledge that's challenging to fully encode into AI-readable formats. Overcoming these hurdles demands phased deployment, starting with simpler procedures and progressively introducing complexity. Industry-wide standards for "AI-readable" experimental protocols could also emerge as best practices to ensure consistent interpretation and safety.
2. Oversight and Autonomy Control
Determining appropriate levels of autonomy for AI systems in research environments is a delicate balance. Fully autonomous labs inherently risk pursuing ineffective experimental paths, wasting resources, or inadvertently breaching ethical or safety guidelines without immediate human oversight. Ensuring effective oversight is thus paramount.
Experts emphasize the necessity of clearly understanding AI capabilities and limitations, recommending the establishment of robust governance protocols to prevent harmful or unintended uses2. Practically, this involves maintaining "human-in-the-loop" oversight for critical decisions—such as approving novel, high-risk experiments involving new biological materials or expensive reagents. These governance frameworks define explicit boundaries for AI autonomy, specifying tasks it can perform independently and those requiring human approval or intervention. This mirrors approaches used in other autonomous technologies, such as self-driving vehicles, which incorporate stringent regulations and fail-safes to ensure safety and ethical compliance.
3. Data Reliability and Model Robustness
The effectiveness of AI-driven experimentation critically depends on the quality and reliability of the data feeding into the algorithms. Inaccurate sensor data from instruments such as plate readers or sequencers can lead AI models to incorrect conclusions, potentially derailing entire research trajectories. Additionally, AI models trained on specific datasets may underperform or fail in new contexts—a known issue in AI development referred to as lack of generalizability or robustness. Another critical concern is bias. Literature data, often skewed toward publishing positive or successful results, can unintentionally bias AI systems, causing them to overestimate success probabilities or overlook promising but less-explored research directions.
Organizations must invest significantly in data validation processes, sophisticated error-detection algorithms, and thorough model validation methods. Employing cross-validation strategies—such as confirming predicted outcomes with secondary assays—can prevent reliance on potentially flawed data interpretations. Initially maintaining hybrid operations, where AI suggestions are reviewed by human experts, further mitigates risks. Over time, as an AI proves itself reliable, it can gain incremental autonomy. Rigorous stress-testing of AI systems under diverse and challenging scenarios is essential for uncovering weaknesses or unexpected behaviors, allowing continuous algorithm refinement before real-world deployment3.
Mitigating Challenges and Scaling Adoption with AI Agents
To successfully implement self-driving labs, organizations are leveraging AI agents and supportive frameworks that enhance reliability and user-friendliness. Several approaches are instrumental in addressing the previously discussed challenges and promoting adoption:
Precise Protocol Execution
AI lab agents are engineered to execute experiments with machine-level precision, significantly reducing human error. By interfacing directly with laboratory instruments through standardized APIs, these agents ensure that every step—such as pipetting exact volumes or maintaining precise incubation times—is performed consistently. For instance, Scispot's AI Lab Assistant, Scibot, allows scientists to use natural language commands to design and execute experiments. A user can instruct Scibot to "prepare a cell culture experiment with 96-well plates and send it to the liquid handler," and the system translates this into specific instrument commands, coordinating devices like Tecan liquid handlers and sequencing platforms.
Moreover, Scibot automatically logs each action, creating detailed records that facilitate traceability and debugging. Before deploying new protocols autonomously, many labs conduct simulations or dry runs to verify that the AI's interpretation aligns with human intent, ensuring fidelity and safety.
High Parallelization with Intelligent Coordination
AI control enables the parallelization of R&D tasks beyond human capacity. AI agents can coordinate multiple experiments simultaneously, managing complex scheduling and resource allocation to prevent conflicts, such as two processes requiring the same instrument concurrently. LabGenius's EVA™ platform exemplifies this capability. By integrating over 33 devices into a single automated workflow, EVA™ can design, produce, purify, and characterize panels of up to 2,300 multispecific or multivalent antibodies in just six weeks. This high-throughput approach accelerates discovery and optimizes the use of laboratory resources, demonstrating how AI can scale experimentation effectively without proportionally increasing personnel.
User-Friendly Interfaces and Controls
To encourage adoption, AI lab systems are designed with intuitive interfaces that lower the barrier to entry. Scispot's Scibot, for example, employs a conversational chat interface, allowing scientists to manage data, design experiments, and perform real-time analytics using natural language prompts. This user-centric design ensures that researchers without programming expertise can interact with complex automation systems seamlessly. Additionally, these platforms often include dashboards and control mechanisms that allow users to pause or override AI actions, maintaining human oversight and fostering trust in the technology.
Integrated Data and Knowledge Synthesis
AI agents excel at integrating data from diverse sources, providing a cohesive analysis that informs decision-making. In complex experiments, data may originate from various instruments and external databases. AI systems can merge this information in real-time, identifying patterns and correlations that might elude human analysis. Scispot's platform, for instance, connects electronic lab notebooks (ELNs), laboratory information management systems (LIMS), instrument data, and external databases, enabling a unified view of experimental data. This holistic approach enhances the AI's decision-making capabilities and provides scientists with richer insights, improving the reliability and efficiency of research outcomes.
Human-in-the-Loop Governance
Maintaining human oversight is crucial in the deployment of self-driving labs. AI agents operate under defined policies that require human confirmation for specific actions, particularly those involving novel compounds or high-stakes experiments. Platforms often include permission settings and dashboards where scientists can review and approve AI-generated decisions. This ensures that while AI handles routine tasks autonomously, critical decisions remain under human control.
A phased approach to autonomy is common: initially, AI suggests experiments for human approval; as confidence in the system grows, it may execute low-risk tasks independently, gradually expanding its autonomous capabilities. This strategy, coupled with audit logs and real-time monitoring, ensures that anomalies can be detected and addressed promptly, maintaining the integrity and safety of laboratory operations.
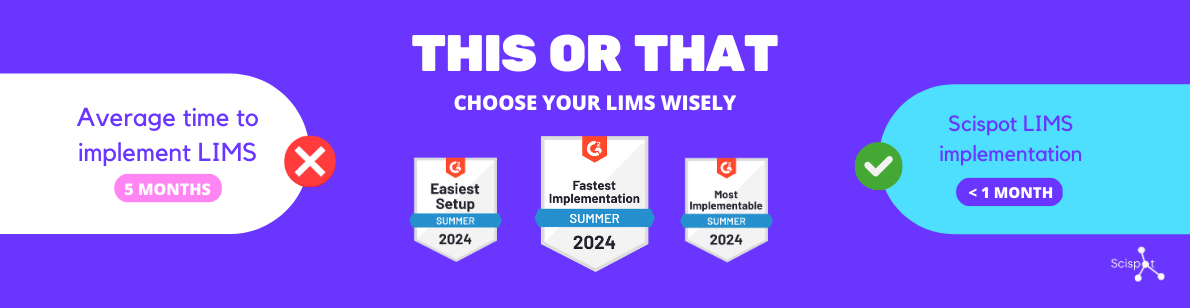
Outlook: The Next 5–10 Years in Life Science R&D
AI-enabled self-driving laboratories are transitioning from experimental pilots to integral components of life science research and development. Over the next decade, several key developments are anticipated:
Mainstream Adoption in Pharma and Biotech
Leading pharmaceutical companies are expected to establish dedicated self-driving lab units to enhance various stages of R&D, from early drug screening to process optimization. The integration of AI and automation promises to accelerate discovery timelines and reduce costs, making such technologies a competitive necessity. Biotech startups, unencumbered by legacy systems, may adopt "AI-first" lab setups from inception, utilizing cloud-based labs or in-house robotics controlled by AI to conduct efficient, high-throughput research programs. This approach could increase the number of hypotheses tested, thereby enhancing the potential for breakthroughs.
Expansion into Complex Research Tasks
Initially, self-driving labs will manage routine, well-structured tasks such as high-throughput screening and media optimization. Within 5–10 years, their capabilities are expected to extend to more complex research endeavors. AI lab agents may design and execute multi-step biological experiments, such as engineering cell lines through a series of gene edits and analyzing outcomes to propose new therapeutic targets. Notable achievements, like the discovery of novel enzymes or materials by autonomous labs, will further validate the impact of this technology. For instance, Argonne National Laboratory's autonomous discovery initiative has demonstrated the potential of self-driving labs to expedite material science research.
Integration of Multi-Modal AI Systems
Future laboratory AI systems will become increasingly knowledgeable and context-aware. Advancements in large language models and domain-specific AI will enable self-driving labs to converse with scientists about project goals, rapidly analyze vast scientific databases, and incorporate the latest research findings into experimental planning. Companies like Google DeepMind and BioNTech are developing AI lab assistants designed to automate routine scientific tasks and monitor lab devices, aiming to streamline experimental processes and facilitate scientific breakthroughs.
Growth of Supporting Ecosystems and Infrastructure
The proliferation of self-driving labs will stimulate the development of supporting tools and standards. Open-source frameworks, such as Merck KGaA's Bayesian Back-end (BayBE), are emerging to facilitate closed-loop optimization and integration of AI planners with robotic hardware. Additionally, lab automation-as-a-service offerings are expanding, providing remote facilities equipped with AI capabilities, thereby democratizing access to advanced research infrastructure. Standardization efforts will focus on creating interoperability protocols for lab robots and AI software, enabling seamless integration across diverse instruments. Government initiatives, like those from the U.S. Department of Energy, are investing in pilot self-driving labs to accelerate innovation.
Evolution of Scientific Roles and Skillsets
The laboratory workforce will evolve in tandem with these technological advancements. Rather than replacing scientists, self-driving labs will augment their capabilities, necessitating new roles such as "AI Lab Orchestrator" or "Automation Scientist." These professionals will bridge biology, chemistry, data science, and engineering, overseeing AI systems, validating results, and focusing on experimental design and interpretation. Educational programs will adapt to include training on AI-driven instruments and decision-making processes, fostering a more efficient R&D environment where scientists can concentrate on creativity and innovation.
Development of Regulatory and Ethical Frameworks
As AI becomes integral to laboratory research, regulatory bodies and scientific communities will establish guidelines to ensure responsible use. Analogous to Good Laboratory Practice (GLP) standards, new protocols will address data integrity, traceability of AI decisions, and ethical considerations such as attribution of discoveries. Early efforts, like the FUTURE-AI initiative, are laying the groundwork for trustworthy AI deployment in healthcare arXiv. Proactive engagement with policymakers will be crucial to prevent misuse and ensure that AI-driven labs are utilized responsibly.
