As the integration of biology and AI deepens, managing laboratory data efficiently has become a fundamental necessity. The traditional scientific data management system (SDMS) was designed to store vast amounts of research data, but it often lacks the necessary tools for real-time analysis, collaboration, and automation. Many legacy SDMS platforms require extensive manual input, leading to inefficiencies and bottlenecks in the research process., once seen as a solution, often falls short in providing seamless, actionable insights. Instead of being dynamic engines for discovery, outdated scientific data management systems have turned into passive data repositories, leaving valuable research insights untapped.
With biotech advancements generating vast datasets daily—ranging from genomic sequences to high-throughput screening results—labs require scientific data management systems that not only store but also contextualize and analyze data. Without this capability, researchers face challenges in data duplication, accessibility, and standardization, which hinder collaboration and slow innovation.
The Evolution of Scientific Data Management System Software
Legacy scientific data management system software primarily focused on storing and archiving data, offering limited interoperability with modern analytical tools. However, as biotech labs increasingly rely on AI, automation, and cloud computing, these older solutions fail to keep up with the demands of fast-paced research environments. Modern scientific data management systems must go beyond basic storage to actively enable data-driven discoveries. For example, they should integrate machine learning capabilities, allowing researchers to detect patterns and anomalies in datasets that might otherwise go unnoticed. Additionally, a well-structured SDMS should support AI-powered data enrichment to provide deeper insights from raw experimental data. and actively organize, standardize, and integrate data from multiple sources, ensuring it is Findable, Accessible, Interoperable, and Reusable (FAIR).
Challenges in Traditional Data Management Approaches
- Fragmented Data Silos: Labs often use multiple disconnected tools, leading to difficulties in retrieving, integrating, and analyzing data across projects.
- Lack of Automation: Manual data entry and retrieval slow down research and increase the risk of errors.
- Incompatibility with AI & ML: Many legacy systems cannot process or structure data in ways that make it useful for machine learning models.
- Limited Scalability: As research grows, many SDMS struggle to handle increasing data volumes and diverse experimental workflows.
Key Features of a Next-Gen Scientific Data Management System (SDMS)
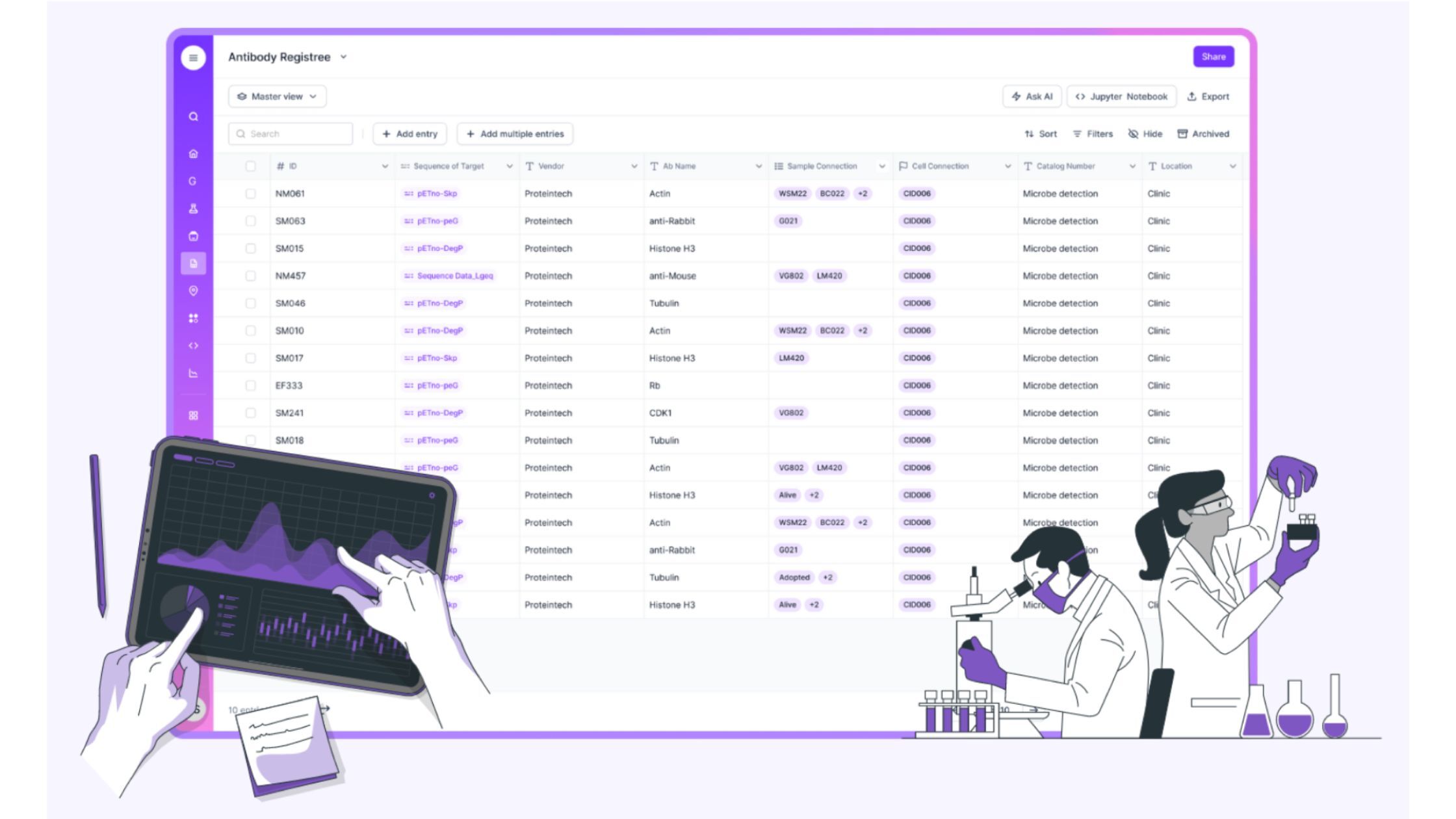
Scispot's API-first alt-SDMS is the most intuitive, customizable, and fast-implementing Scientific Datalake.
1. Intelligent Data Standardization and FAIR Compliance
Traditional scientific data management systems often leave data scattered, unstructured, and difficult to access. A modern scientific data management system SDMS applies FAIR principles to ensure that data is structured, searchable, and reusable. This enables biotech firms to extract meaningful insights, automate workflows, and enhance reproducibility in experiments. For example, Scispot enables seamless metadata tracking, eliminating inconsistencies and making research data more reliable.
Additionally, compliance with industry standards such as ISO 17025, GLP (Good Laboratory Practices), and FDA 21 CFR Part 11 is crucial in regulated environments. A modern scientific data management system SDMS ensures compliance through audit trails, role-based access, and automated reporting, reducing the burden on researchers while enhancing data security.
A leading molecular diagnostics company reduced data errors by 90% and improved efficiency by 80% using Scispot’s alt-SDMS, enabling seamless standardization and AI-ready insights.
2. Customization and Scalability
Most traditional scientific data management system software offers rigid structures that don’t adapt to the unique needs of different research fields. Scispot provides customizable workflows, allowing biotech labs to build data pipelines that fit their specific needs, from genomics to immunotherapy. Whether a lab is tracking patient-derived xenografts or integrating CRISPR workflows, Scispot adapts without requiring extensive software modifications.
Scalability is essential for growing biotech firms, as research projects generate exponentially increasing datasets. Many firms struggle with managing multi-terabyte-scale data while maintaining performance efficiency. A robust SDMS should offer dynamic resource allocation, cloud-based storage expansion, and automated data partitioning to handle high-throughput workflows seamlessly.. Scispot’s cloud-based SDMS ensures that as data volumes grow, processing speeds remain high, storage remains secure, and research teams can collaborate without performance bottlenecks.
3. Seamless Integration with Lab Tech Stacks
A true next-generation scientific data management system integrates with all major LIMS, ELN, and bioinformatics platforms, ensuring that data flows seamlessly between laboratory instruments, computational tools, and analysis software. Scispot stands out by offering deep integrations with high-throughput sequencing machines, cloud-based AI analytics, one-click integration and real-time collaboration tools, ensuring scientists spend less time on data wrangling and more on innovation.
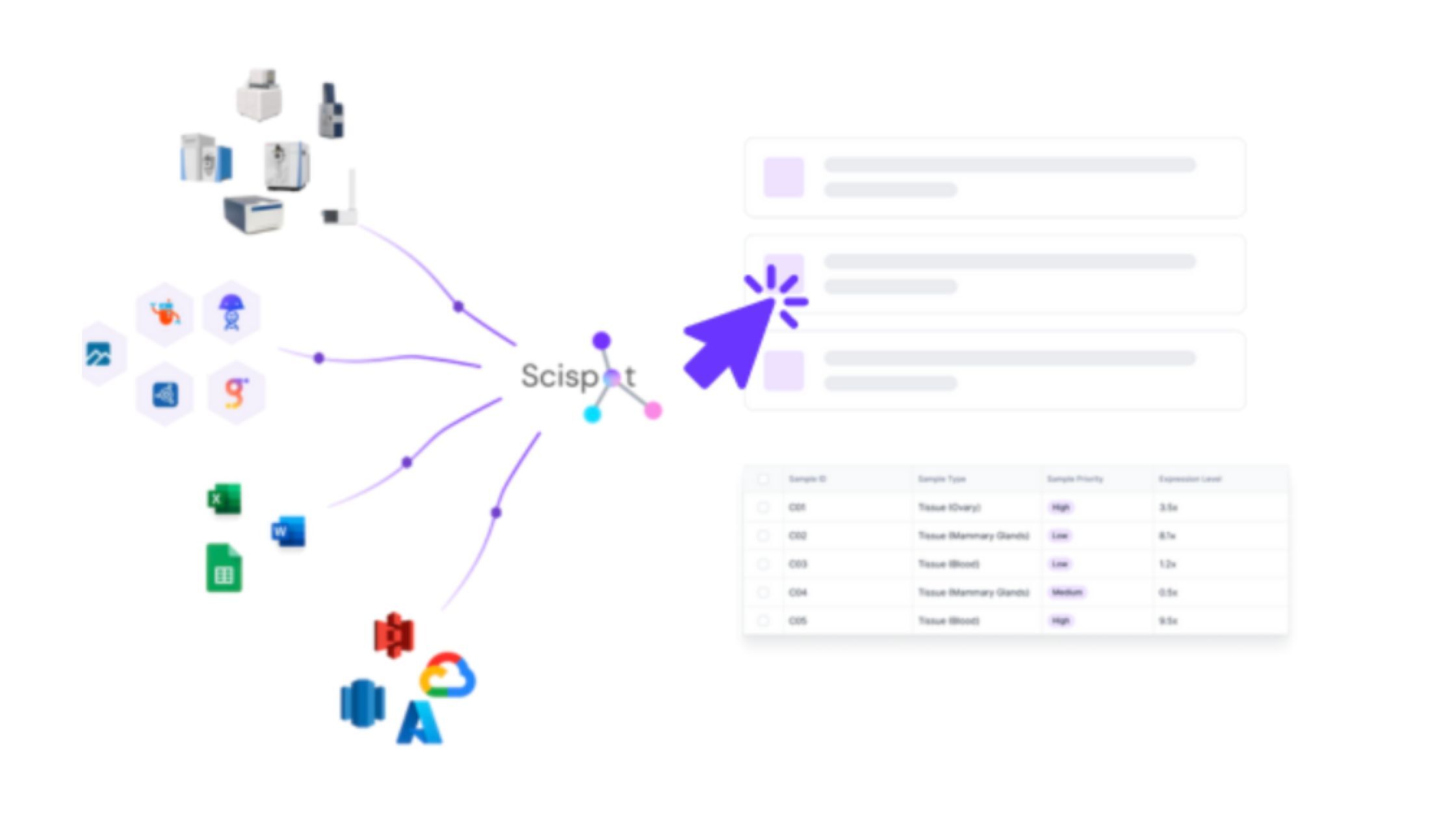
One-click lab integration to effortlessly centralize and standardize your data formats for seamless analysis, machine learning, and AI with Scispot GLUE
Moreover, automation tools embedded within scientific data management system software reduce repetitive tasks by implementing robotic process automation (RPA) and intelligent data pipelines. For instance, Scispot automates real-time data parsing, metadata tagging, and anomaly detection, significantly cutting down manual effort and improving data quality.. For instance, Scispot automates data ingestion from lab instruments, eliminating the need for manual data transfer and reducing errors. By integrating with platforms such as AWS, Google Cloud, and Azure, Scispot ensures researchers can access and analyze data from anywhere in real time.
A Cancer Research Institute and improved cross-team collaboration by 60% with Scispot’s alt-SDMS, eliminating silos and accelerating research workflows.
Why Scispot is the Future of Scientific Data Management Systems
Scispot’s scientific data management system SDMS is not just a data repository—it’s a dynamic, AI-powered platform that transforms lab data into a strategic asset. It unifies fragmented data, eliminates inefficiencies, and empowers biotech labs with automation, real-time analytics, and streamlined collaboration.
What sets Scispot apart?
- End-to-end laboratory automation: From data capture to reporting, Scispot streamlines every aspect of research.
- AI-powered insights: Advanced analytics allow scientists to predict trends, detect anomalies, and make data-driven decisions faster.
- Security & Compliance: Built-in encryption, version control, and audit logs ensure that sensitive research data is always protected.
- Cross-team collaboration: Cloud-native design enables researchers, CROs, and partners to collaborate efficiently in a single platform.
By moving beyond static databases, Scispot provides a smarter way to manage, analyze, and unlock research data. Unlike conventional SDMS platforms that function as data warehouses, Scispot integrates AI-powered data curation, cross-experimental correlation, and predictive analytics, enabling biotech firms to accelerate drug discovery and personalized medicine research. the full potential of scientific data. Whether it's for early-stage biotech startups, contract research organizations (CROs), or large-scale pharma R&D, Scispot offers a future-proof solution for scientific data management.
Want to see how Scispot's scientific data management system can transform your lab? Book a demo today.
