Biotech organizations are increasingly recognizing the critical role data plays in decision making. The principle of FAIR - Findable, Accessible, Interoperable, and Reusable - is the ideal standard. However, balancing widespread empowerment of scientists with data governance often leads to an unexpected quandary. On the one hand, granting scientists broad access can result in duplicated experiments and redundant results. On the other hand, rigorous data governance introduces processes and standards that may cause substantial backlogs in data model construction.
Building data model is a balancing act between scientists empowerment & data governance

Existing LIMS (Laboratory Information Management Systems) and ELNs (Electronic Laboratory Notebooks) often tilt the balance towards one extreme or the other. These systems fall short in providing end users with the capacity to construct data models that are easily configurable yet comply with requisite processes and standards.
As a result most ELN and LIMS end up being data graveyards. Scientist end up storing their notebook entries, sample metadata and structured data models in variety of formats, schema and systems.
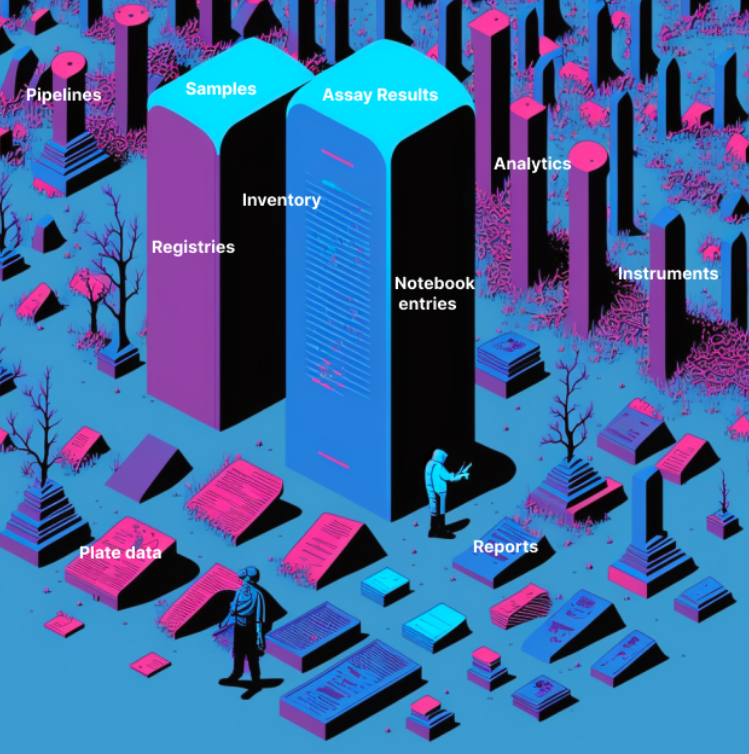
Airtable and Notion exemplify one end of the spectrum, empowering scientists but failing to ensure FAIR standards. Conversely, traditional LIMS systems enforce stringent processes but lack flexibility and scalability as companies grow. These LIMS are not designed to function as adaptable data warehouses.Cloud data warehouses, such as Snowflake, BigQuery, Redshift, and Databricks, offer an alternative. They provide a single, rapidly accessible source of truth, easy to manage and scalable enough for use by analysts and non-technical users alike. Nonetheless, they are not intrinsically designed with the biotech context in mind.
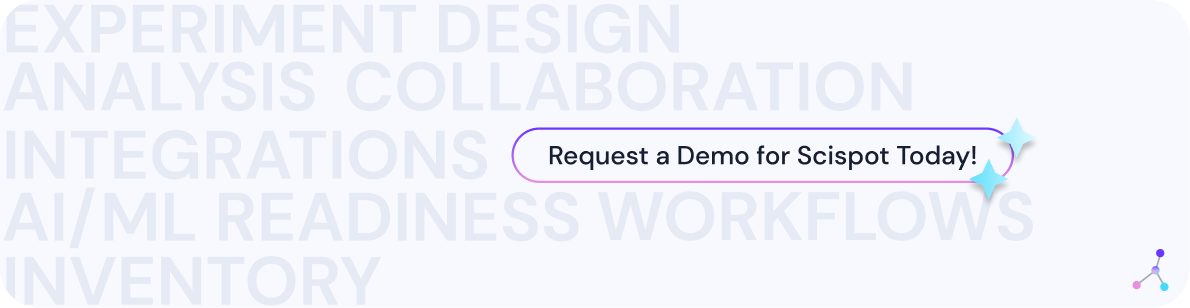
The challenge for organizations utilizing data has always been finding the equilibrium between user empowerment and organizational control. The implementation of FAIR data models necessitates a careful balance between data governance and the freedom to design personalized data dictionaries.
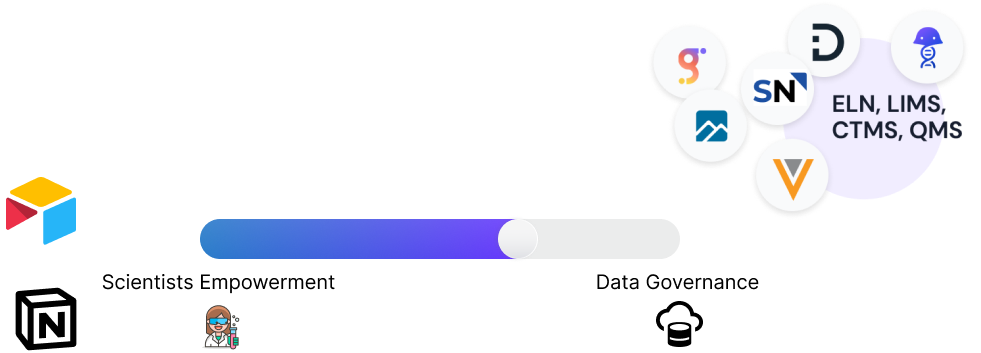
In this respect, every organization needs a blend of a thoroughly vetted data model structure and the freedom for individuals to explore beyond these limits. Investment in data model construction for one-off queries is often seen as a misuse of resources.
Scispot is addressing this challenge head-on. The platform provides an orchestration engine that enables end users to design their data models using templates of a vetted model. Its APIs maintain a flat structure, offering the flexibility to design data models for any schema.
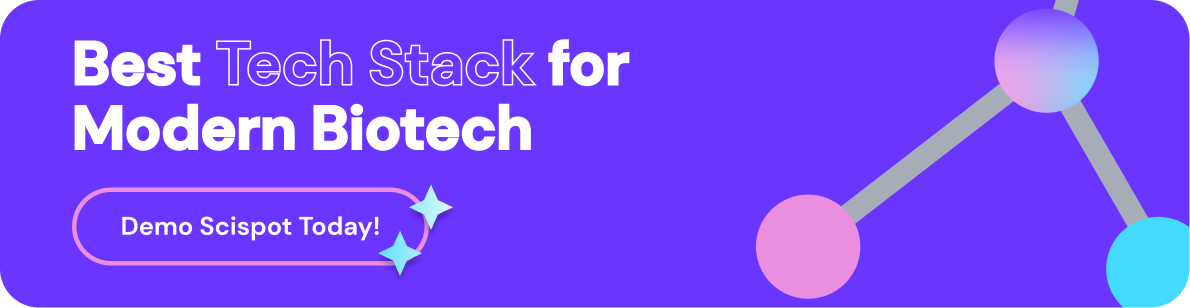
Thus, Scispot serves as a call to action: transcend the limitations of traditional LIMS that are stunting your data models. Embrace the freedom of flexible, scalable, and context-specific data modeling, allowing your organization to effectively leverage data for critical decision making.
Design your data model using Scispot API
Design your data model using Scispot Labsheets
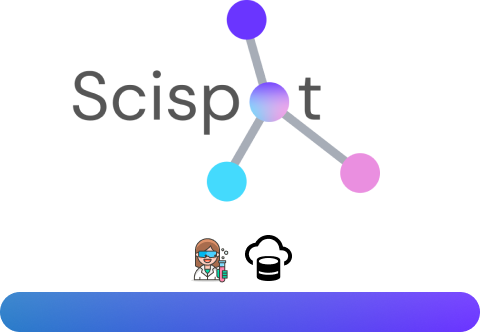