Biotech R&D is notoriously expensive. Bringing a single drug to market takes 10–15 years and costs over $2.6 billion, according to the Tufts Center for the Study of Drug Development. Yet, the return on investment (ROI) often remains frustratingly low. With only 10% of drug candidates successfully making it from preclinical stages to FDA approval, inefficiencies in discovery, development, and manufacturing continue to drain resources.
Delays in clinical trials can cost companies $600,000 to $8 million per day, driven by high operational costs, extended timelines, and lost opportunities for revenue. Each additional day of delay increases pressure on budgets and slows the availability of critical treatments. Manufacturing bottlenecks add another layer of expense, as issues like batch variability or production inefficiencies can lead to costly rework or product losses. These challenges aren’t just financial—they hinder innovation and delay life-saving therapies from reaching patients in need.
Enter AI agents—autonomous systems designed to analyze, reason, and act. These intelligent tools promise to tackle inefficiencies, reduce costs, and accelerate breakthroughs. But can they deliver the transformative impact biotech needs?
What Are AI Agents for Biotech?
AI agents, such as those developed by Scispot, are intelligent systems built to manage complex, multi-step workflows in biotech. Unlike traditional AI, which focuses on specific tasks, AI agents work dynamically by integrating real-time data, learning from interactions, and adapting to new challenges. This flexibility makes them ideal for streamlining various lab and research processes.
In drug discovery, AI agents can process extensive datasets from high-throughput screenings to identify compounds with the highest potential for success in preclinical trials. These agents analyze millions of compounds, prioritizing those with strong efficacy while flagging others with undesirable properties, such as toxicity. By automating these processes, AI agents enable researchers to concentrate on the most promising candidates, significantly reducing time and resource requirements. For example, Scispot’s AI agent, Plato, seamlessly integrates with liquid handlers and plate readers to automate tasks like sample allocation, assay result analysis, and real-time quality control checks, ensuring a streamlined and efficient workflow.
In biomanufacturing, AI agents can monitor critical production parameters like temperature, pH, and pressure, identifying deviations that might affect product quality. They can also adjust settings autonomously to maintain consistency. Imagine a monoclonal antibody production process where the AI agent detects a drop in dissolved oxygen levels in a bioreactor and adjusts the aeration rates to ensure optimal cell growth conditions.
AI agents also improve efficiency in clinical trials by analyzing patient data to identify risks and recommend adjustments. They can predict dropout risks and optimize trial protocols to improve outcomes. For example, by integrating data from patient monitoring systems, a Scispot AI agent can detect trends in biomarker changes and alert researchers to potential adverse reactions, allowing for timely protocol modifications without delaying the trial.
Diagnostics labs can also benefit significantly from AI agents as well. They analyze complex molecular data, automate report generation, and ensure compliance with regulatory standards. For example, in a molecular pathology lab, AI agents can integrate data from qPCR instruments, normalize Ct values, and generate reports highlighting trends in gene expression.
Beyond these applications, AI agents excel at handling advanced data workflows and quality control. They can seamlessly integrate with lab systems like liquid handlers, plate readers, and spectrometers. For instance, in a lab working on CRISPR gene edits, a Scispot AI agent could ingest raw data from a plate reader, compare fluorescence intensity with controls, and flag samples with off-target effects for further investigation.
In crop science, AI agents manage high-throughput screening workflows, optimize plate layouts, and analyze results to identify promising traits like drought resistance or improved yield. An agent might assign controls to a 96-well plate, direct a liquid handler to load samples, and analyze imaging data to determine germination rates or other key metrics.
AI agents combine real-time data processing with adaptive decision-making to transform biotech workflows. Their ability to integrate seamlessly with lab instruments, automate routine tasks, and deliver actionable insights helps labs work more efficiently and achieve better results. Whether it's drug discovery, manufacturing, diagnostics, or agriculture, Scispot AI agents enable labs to do more with their data while reducing manual bottlenecks.
Trailblazers: AI Agents Shaping the Future of Biotech and Beyond
AI agents are driving innovation across industries, offering inspiration for the next generation of biotech applications.
At Johnson & Johnson, an AI agent is transforming drug discovery by optimizing the solvent-switching process. This critical step, used to crystallize molecules during synthesis, traditionally required weeks of manual testing to adjust reaction variables like temperature. By automating this process, the AI agent has significantly reduced timelines and costs while maintaining reliability and quality.
In biomanufacturing, Scispot, in collaboration with two of its biotech partners, is developing a specialized AI agent to tackle batch variability and optimize production workflows. This agent monitors real-time production data—such as temperature, pH, and nutrient levels—while analyzing historical datasets to identify factors that impact yield and product quality. Small inconsistencies in bioreactor conditions, for example, can lead to costly batch failures. By proactively addressing these issues, the agent ensures consistent quality, reduces variability by 15–20%, and improves manufacturing efficiency, particularly during scale-up.
While not specific to biotech, eBay’s agent framework demonstrates the potential of orchestration. By coordinating multiple AI models for tasks like coding and marketing, it learns and adapts over time. A similar framework in biotech could enable AI agents to design experiments, analyze complex datasets, and manage workflows autonomously, significantly boosting lab productivity.
Deutsche Telekom’s "askT" AI agent showcases the value of conversational systems. This "ask me anything" agent handles employee queries and automates administrative tasks like submitting vacation requests. A similar agent in biotech labs could assist researchers by updating protocols, tracking inventory, or troubleshooting experimental errors through a simple chat interface, saving valuable time.
At Cosentino, a "digital workforce" of AI agents manages customer service tasks, allowing human staff to focus on strategic initiatives. In biotech, comparable agents could handle regulatory compliance, automate reporting, or curate data for submission to regulatory bodies, enabling researchers to dedicate more time to discovery and innovation.
These trailblazers highlight how AI agents combine autonomy, adaptability, and intelligence to reshape workflows. In biotech, they are setting the stage for smarter, faster, and more impactful breakthroughs, inspiring the future of intelligent systems in laboratories and beyond.
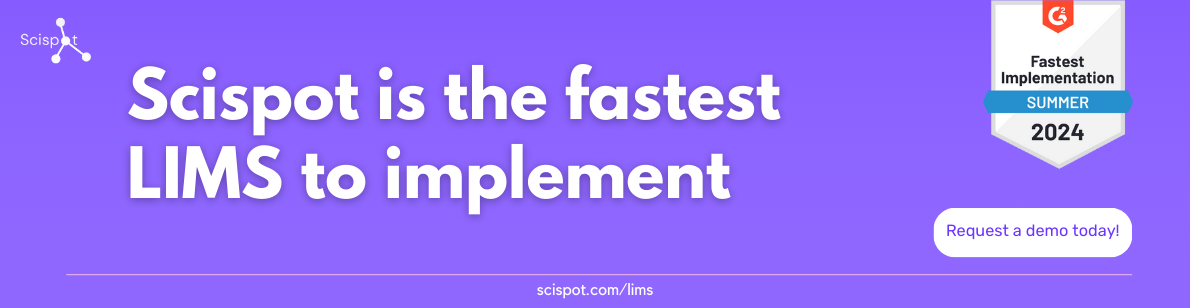
How AI Agents Are Already Helping Biotech
1. Accelerating Drug Discovery
Screening millions of compounds is slow, error-prone, and costly. AI agents like Scispot’s Analytico process vast chemical libraries in hours, flagging the most promising candidates. Atomwise, for example, used AI to screen 68 billion molecules for COVID-19 treatments, achieving results in weeks—a process that would traditionally take years.
2. Reducing Batch Variability in Manufacturing
Small inconsistencies in manufacturing can lead to failed batches and massive losses. Novartis used AI agents to increase batch yields by 15%, while Deloitte estimates that AI-driven manufacturing can reduce waste by 30–50%, saving millions annually.
3. Improving Clinical Trials
Clinical trials represent a significant portion of R&D expenses, often accounting for up to 60% of total costs. AI agents play a pivotal role in reducing these expenses by analyzing patient data, predicting dropout risks, and refining trial protocols to improve efficiency. For example, Accenture has highlighted that AI applications in healthcare have the potential to save the U.S. healthcare industry $150 billion annually by 2026. Additionally, companies like Bristol Myers Squibb have reported using AI to accelerate drug discovery and reduce clinical trial timelines by nearly two years, showcasing AI's ability to optimize trial processes and deliver substantial cost savings.
How AI Agents Improve ROI
AI agents enhance ROI in biotech by addressing the most resource-intensive aspects of R&D. Cutting drug discovery timelines, even slightly, leads to significant cost savings. For example, reducing discovery timelines by 30% can save companies millions per drug by accelerating the path to market and reducing operational expenses. By automating labor-intensive tasks like compound screening or workflow optimization, AI agents make these savings achievable by decreasing manual effort and improving efficiency.
AI agents also minimize costly late-stage failures, which account for nearly 75% of R&D expenses. They achieve this by analyzing early-stage data to identify risks such as poor efficacy, safety concerns, or high dropout risks in clinical trials. By flagging these issues earlier in the development process, companies can pivot or halt projects before committing extensive resources to unsuccessful candidates.
Another key benefit is scalability. Human researchers are limited by time and capacity, whereas AI agents can process millions of datasets and run experiments simultaneously. This allows labs to expand the scope of their research, handling larger-scale projects without proportionally increasing costs. By scaling workflows and reducing failure risks, AI agents enable biotech companies to achieve more with their resources, significantly improving ROI.
Why This Matters
Behind every dataset is a patient waiting for a cure. Every delay means treatments take longer to reach those in need, costing lives, especially in areas like cancer and rare diseases. AI agents like Proto and Plato don’t just make labs faster—they free researchers to focus on innovation, accelerating the development of life-saving treatments.
The ROI isn’t just financial. Faster workflows mean earlier diagnoses, quicker drug approvals, and therapies delivered sooner to patients who depend on them. By bridging the gap between data and discovery, AI agents ensure that biotech advances aren’t just efficient—they’re deeply impactful, saving lives and delivering hope.
The Future of AI Agents
By 2030, 50% of drug discovery tasks could be automated or augmented by AI, fundamentally changing how biotech operates. These agents will drive advancements in personalized medicine by analyzing patient genomes, biomarkers, and health data to create therapies tailored to individual needs. This shift will improve outcomes and reduce side effects, moving beyond the one-size-fits-all approach to treatment.
AI agents will also take on the heavy lifting in R&D workflows. From designing experiments to optimizing clinical trials, they will manage entire pipelines, making real-time adjustments to save time and resources. This will allow researchers to focus on high-level strategies while agents ensure efficiency and precision throughout the process.
Collaboration between labs across the globe will become seamless as AI agents harmonize datasets and facilitate real-time data sharing. This will accelerate research, especially in areas like rare diseases, where fragmented data has traditionally been a barrier to progress. Researchers in different countries will be able to work on the same project, with agents ensuring consistency and compatibility.
Beyond the lab, AI agents will transform manufacturing and supply chains. They will monitor production conditions, predict maintenance needs, and optimize logistics to prevent disruptions. For example, agents could ensure vaccines are transported without spoilage, reducing waste and improving patient access.
In the future, scientists will focus on creativity and innovation as AI agents handle repetitive tasks. These agents could also mentor the next generation of researchers by simulating experiments and teaching advanced concepts. Together, humans and AI agents will create a biotech ecosystem that is faster, smarter, and more precise, delivering life-saving treatments to patients around the world.
Challenges Ahead
The adoption of AI agents in biotech is not without its challenges. One major hurdle is the lack of integrated, high-quality data pipelines in many labs and manufacturing facilities. For AI agents to function effectively, they need access to clean, comprehensive datasets, yet much of the industry still relies on fragmented systems that hinder seamless data flow. For instance, siloed legacy systems in biomanufacturing often prevent AI agents from analyzing real-time production data alongside historical datasets, limiting their ability to optimize workflows.
Transparency is another critical issue. AI agents must provide clear, auditable reasoning behind their recommendations. For example, if an agent suggests halting a clinical trial or adjusting a bioreactor's pH level, researchers and regulators need to understand the decision-making process. Without this explainability, trust in AI systems will remain a barrier, particularly in high-stakes environments like drug development and manufacturing.
Security and ethical concerns further complicate adoption. AI agents processing sensitive patient data or proprietary manufacturing protocols increase the risk of cyberattacks. Additionally, biases in AI training datasets could lead to flawed outcomes, such as overlooking compounds that don’t fit pre-established patterns. Addressing these issues requires rigorous testing, secure infrastructure, and adherence to ethical standards.
Finally, cultural resistance within labs and manufacturing facilities can slow adoption. Transitioning from manual processes to AI-driven systems requires retraining staff and redesigning workflows. Without proper change management, researchers may resist relying on AI agents, fearing loss of control or diminished roles.
Conclusion
AI agents are poised to revolutionize biotech, from optimizing drug discovery timelines to ensuring consistent manufacturing. By enabling faster workflows, reducing failures, and delivering scalable solutions, they provide both financial ROI and the opportunity to bring life-saving treatments to patients faster.
The challenges—data integration, transparency, security, and cultural resistance—are real but surmountable. For example, investing in modern data infrastructure like Scispot, implementing explainable AI frameworks, and fostering collaboration between researchers and technology providers can address these obstacles. The biotech industry cannot afford to wait. With each delay, patients are left waiting for treatments, and opportunities for innovation are lost. AI agents are not just tools—they are essential partners in creating a smarter, more efficient, and impactful future for biotech.
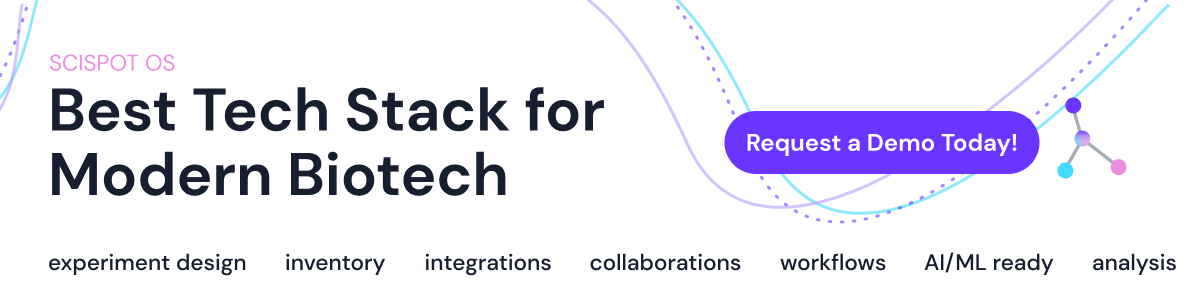